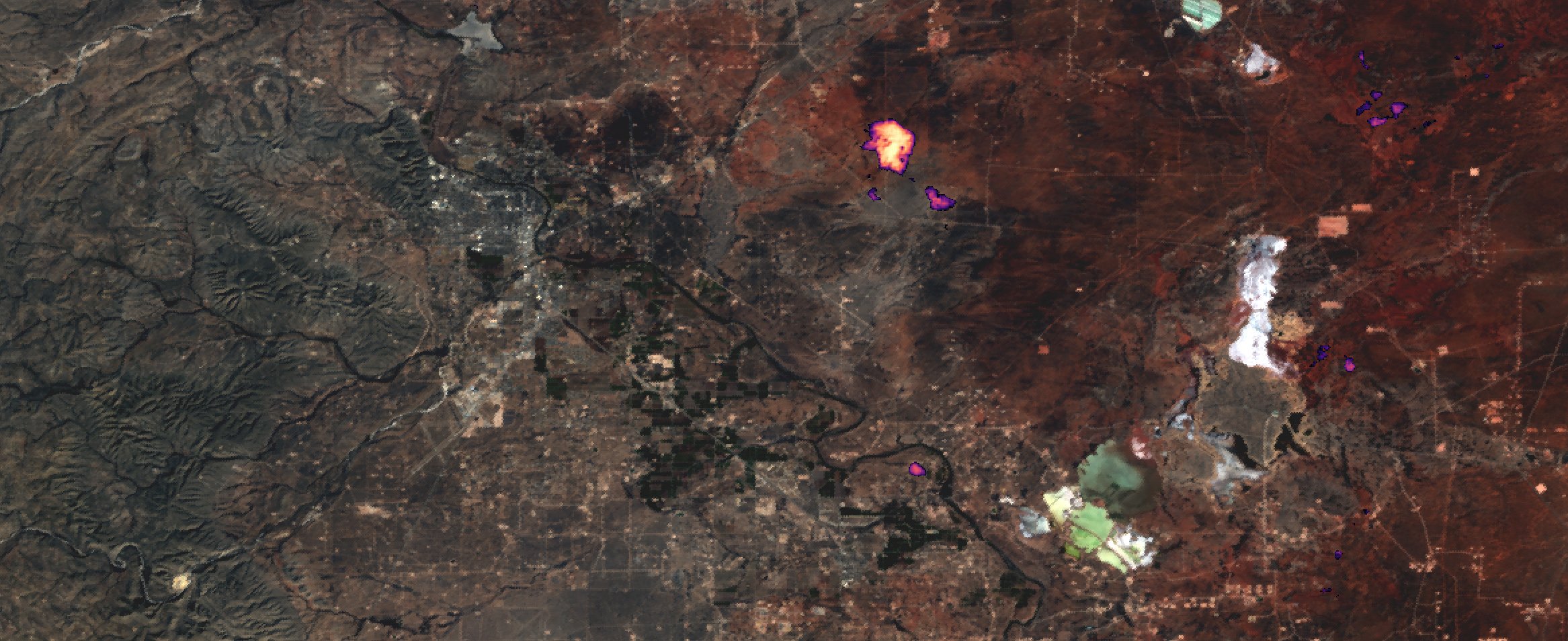
Automated Methane plume monitoring from orbit
Reducing anthropogenic methane emissions is arguably the most urgent lever we have in preventing future catastrophic climate change (UNEP methane assessment report). Among methane emissions, episodic oil and gas super-emissions contribute disproportionately to the concentration of methane in the atmosphere (Lavaux et al 2021). These emissions are caused by equipment failures on oil rigs, pipelines or well pads and are manageable if detected in time.
STARCOP is Trillium's initiative to pair AI methane detection using multiple satellites with diverse detection capabilities to quickly detect methane leaks onboard and provide notifications in near-real-time.
AI pipelines use hyperspectral instruments such as EMIT and AVIRIS to accurately distinguish methane plumes from background noise - previously a problem that required human intervention due to the unique spectral properties of methane. STARCOP was able to detect a recent emission occurred in the Permian basin that was also reported here. Additionally, we also demonstrated detection of large plumes with open multispectral instruments such as Sentinel-2 and WorldView-3.
STARCOP is an accurate and lightweight AI and can be run on edge devices such as the Worldfloods ML payload. STARCOP has been developed with support of the ESA Cognitive Cloud Computing in Space initiative.
Publications
World breakthrough in onboard AI model training presented by Φ-lab at IGARSS
Vít Růžička, Gonzalo Mateo-García, Chris Bridges, Chris Brunskill, Cormac Purcell, Nicolas Longépé, Andrew Markham
Read the bulletin here (published: 7 August 2023)
Brief: At the International Geoscience and Remote Sensing Symposium (IGARSS) on 21 July, 2023, ESA Φ-lab presented the results of groundbreaking research in artificial intelligence (AI) aboard Earth observation satellites. Carried out by Oxford University and Trillium Technologies in collaboration with Φ-lab, the research successfully trained a cloud-detection Machine Learning model while in flight on a D-Orbit ION mission.
CH4Net: a deep learning model for monitoring methane super-emitters with Sentinel-2 imagery
Anna Vaughan, Gonzalo Mateo-García, Luis Gómez-Chova, Vít Růžička, Luis Guanter, and Itziar Irakulis-Loitxate
Read the paper here (published: 3 May 2024)
Abstract: We present a deep learning model, CH4Net, for automated monitoring of methane super-emitters from Sentinel-2 data. When trained on images of 21 methane super-emitters from 2017–2020 and evaluated on images from 2021 this model achieves a scene-level accuracy of 0.83 and pixel-level balanced accuracy of 0.77. For individual emitters, accuracy is greater than 0.8 for 17 out of the 21 sites. We further demonstrate that CH4Net can successfully be applied to monitor two superemitter locations with similar background characteristics not included in the training set, with accuracies of 0.92 and 0.96. In addition to the CH4Net model we compile and open source a hand annotated training dataset consisting of 925 methane plume masks.
Journal Top 100 of 2023
Semantic segmentation of methane plumes with hyperspectral machine learning models
Vít Růžička, Gonzalo Mateo-Garcia,Luis Gómez-Chova,Anna Vaughan,Luis Guanter & Andrew Markham
Read the paper here (published: 17 November 2023)
Abstract: Methane is the second most important greenhouse gas contributor to climate change; at the same time its reduction has been denoted as one of the fastest pathways to preventing temperature growth due to its short atmospheric lifetime. In particular, the mitigation of active point-sources associated with the fossil fuel industry has a strong and cost-effective mitigation potential. Detection of methane plumes in remote sensing data is possible, but the existing approaches exhibit high false positive rates and need manual intervention. Machine learning research in this area is limited due to the lack of large real-world annotated datasets. In this work, we are publicly releasing a machine learning ready dataset with manually refined annotation of methane plumes. We present labelled hyperspectral data from the AVIRIS-NG sensor and provide simulated multispectral WorldView-3 views of the same data to allow for model benchmarking across hyperspectral and multispectral sensors. We propose sensor agnostic machine learning architectures, using classical methane enhancement products as input features. Our HyperSTARCOP model outperforms strong matched filter baseline by over 25% in F1 score, while reducing its false positive rate per classified tile by over 41.83%. Additionally, we demonstrate zero-shot generalisation of our trained model on data from the EMIT hyperspectral instrument, despite the differences in the spectral and spatial resolution between the two sensors: in an annotated subset of EMIT images HyperSTARCOP achieves a 40% gain in F1 score over the baseline.