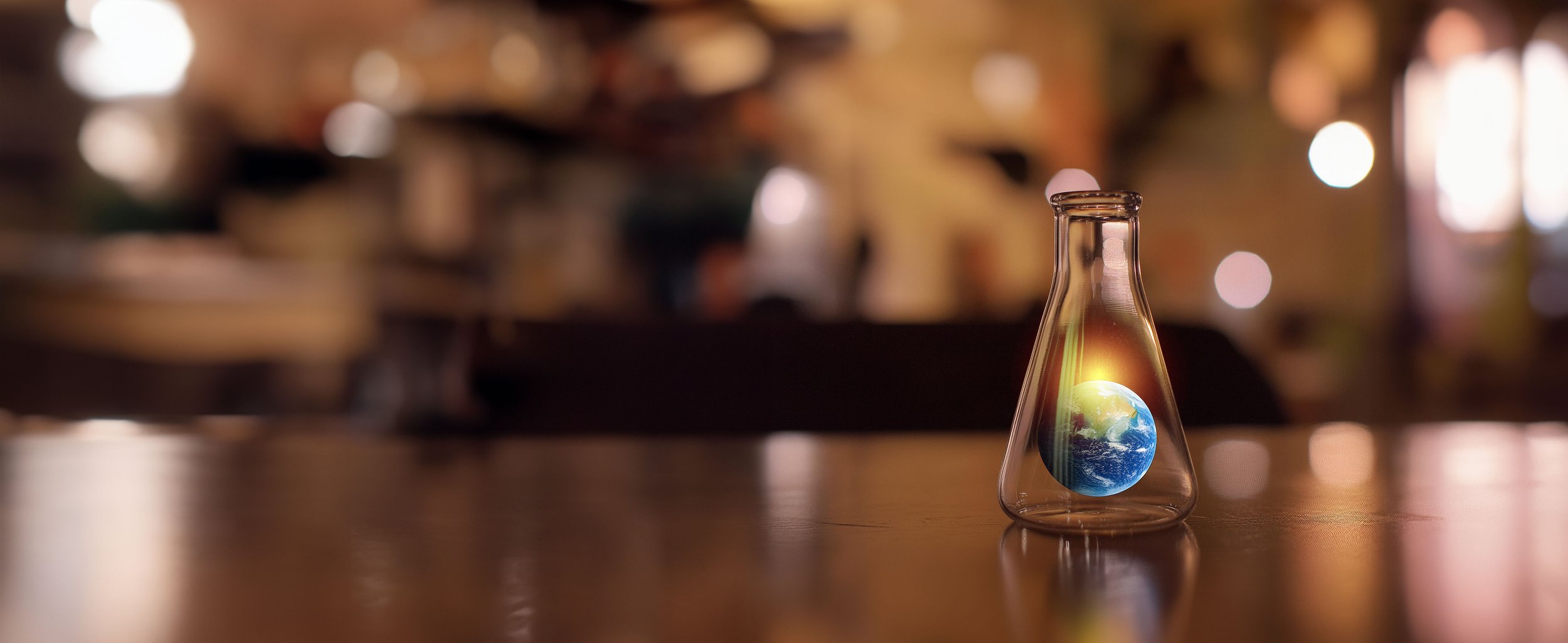
Foundation Models for Science
Foundation models for science potentially represent a paradigm shift in machine learning from single-purpose, supervised machine learning pipelines, to general purpose, self-supervised models running at enormous scales.
Foundation models can:
act as a queryable knowledge store of large volumes of data
democratize the use of AI: enabling faster roll-out of capabilities such as segmentation, classification, object detection, change detection and regression - accessible even to people without coding skills
generalize well: maintain high performance across distinct geographic areas or between time-periods, and
be generative: producing predictions, synthetic data and model phenomena.
Foundation models translate the important information from multiple sensors and simulations into lower-dimensional ‘embeddings’ that are much easier to query, or use to generate new insight-level data, or directly answer questions.
However the journey from raw-data to embeddings requires trade-offs and therefore the way these models are built requires science-led thinking on how to make them genuinely useful tools for discovery and analysis.
Trillium is currently developing two Foundation Models:
SDO-FM (in partnership with NASA and Google Cloud) is a multi-model foundation model of our Sun that aggregates solar magnetic data and multi-band images of the Sun’s atmosphere into the embedding space.
SDO-FM uses the SDOML V2 analysis ready data product. A live inference stream can be viewed here: http://sdomldemo.org/
An overview of SDOML V2 can be viewed here:
SAR-FM (in partnership with the LSA, Lux Provide and ESA) is a foundation model trained using synthetic aperture radar (SAR) data to generalize across geographic regions, for a broad range of downstream applications.
SAR-FM won best paper at the Climatechange.ai workshop at NeurIPS 2023. The paper can be viewed here: https://www.climatechange.ai/papers/neurips2023/76