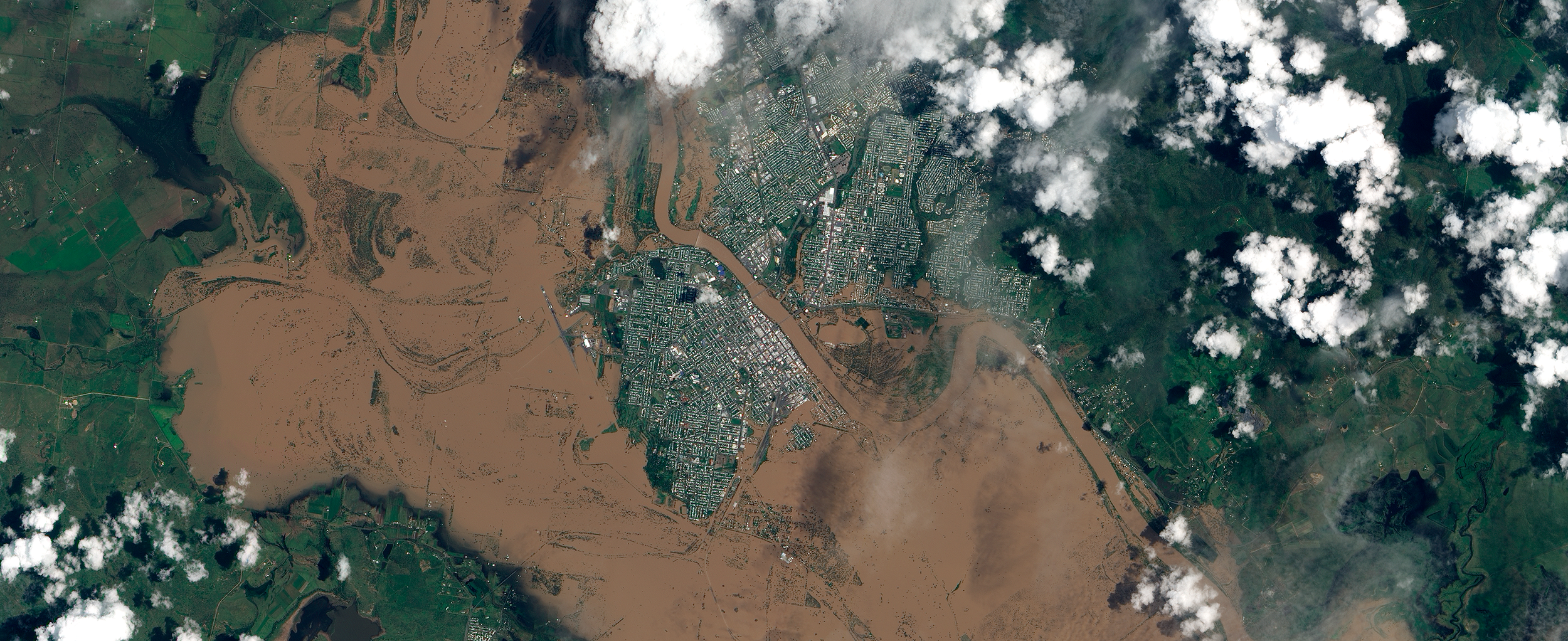
Rapid ML derived flood maps at scale
Floods are the most common natural disaster worldwide, affecting millions of people every year. We need better tools to predict flooding in advance and to support communities through recovery.
During early 2022 almost 2,000 kilometers of Australia’s eastern seaboard was flooded due to prolonged heavy rainfall. The sheer scale of this disaster made mapping the extent highly challenging - even with modern satellite technology. Existing flood-mapping tools struggle to analyse the large volumes of data and simply can’t deliver timely and accurate water extent maps.
Trillium has developed a robust and scalable flood mapping toolkit based on cutting-edge machine learning technology - FloodMapper. We used FloodMapper to generate highly accurate maps of the East Australian floods from satellite imagery in only a few days. Click on the brochure to find out more.
FloodMapper is built using the open ML4FLOODS toolbox , which was developed during Trillium’s Frontier Development Lab program and enhanced with the support of the UK Space Agency.
Flood Maps for the Australian East Coast
Floodmapper.ai select publications
Download the PDF brochure on our FloodMapper system for generating large-scale flood-extent maps from open satellite data.
Global flood extent segmentation in optical satellite images
Enrique Portalés-Julià, Gonzalo Mateo-García, Cormac Purcell & Luis Gómez-Chova
Read the paper here (published: 20 November 2023)
Floods are among the most destructive extreme events that exist, being the main cause of people affected by natural disasters. In the near future, estimated flood intensity and frequency are projected to increase. In this context, automatic and accurate satellite-derived flood maps are key for fast emergency response and damage assessment. However, current approaches for operational flood mapping present limitations due to cloud coverage on acquired satellite images, the accuracy of flood detection, and the generalization of methods across different geographies. In this work, a machine learning framework for operational flood mapping from optical satellite images addressing these problems is presented. It is based on a clouds-aware segmentation model trained in an extended version of the WorldFloods dataset. The model produces accurate and fast water segmentation masks even in areas covered by semitransparent clouds, increasing the coverage for emergency response scenarios. The proposed approach can be applied to both Sentinel-2 and Landsat 8/9 data, which enables a much higher revisit of the damaged region, also key for operational purposes. Detection accuracy and generalization of proposed model is carefully evaluated in a novel global dataset composed of manually labeled flood maps. We provide evidence of better performance than current operational methods based on thresholding spectral indices. Moreover, we demonstrate the applicability of our pipeline to map recent large flood events that occurred in Pakistan, between June and September 2022, and in Australia, between February and April 2022. Finally, the high-resolution (10-30m) flood extent maps are intersected with other high-resolution layers of cropland, building delineations, and population density. Using this workflow, we estimated that approximately 10 million people were affected and 700k buildings and 25,000 km2 of cropland were flooded in 2022 Pakistan floods.